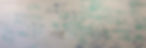
My group works on both foundations of statistical machine learning and applications in biomedicine and healthcare. We develop new technologies that make ML more accountable and reliable in the wild and make novel scientific discoveries.
Much of our ML research is motivated by transformative applications in biotech and health. We collaborate with and advise many companies, including Adela Bio, Amazon, Anthem, Collinear.AI, Enable Medicine, Exai, Fidocure, Genentech, Genmab, Google, Gradio, Greenstone Bio, Intervenn Bio, InVision, Nuclei.io, and Virtusa (several of these companies grew out of our group's research). We are excited to scale and deploy our research with industry partners.
Some active research areas (please see Publications for more):
Accountable AI

We have led research in detecting and reducing harmful biases and stereotypes in AI systems (e.g. NeurIPS'16, Nature'18, PNAS'18, Nature'19, Nature MI'21, Nature Med'21, ICML'22, Science Advances'22). We have also developed some of the first methods to efficiently delete personal data from trained ML models (NeurIPS'19) and to assign algorithmic responsibility (AIES'19). Our methods are used by many companies.
Data-centric AI
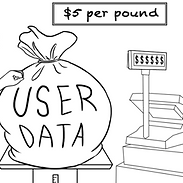

Data limitation is the biggest challenge in applying ML. We have developed Data Shapley to quantify which data is more or less valuable, and to amplify the more useful data (e.g. ICML'19, AISTATS'21, AISTATS'22). We are also working on methods to audit and clean datasets (Nature MI'22).
Combining the best of deep learning and statistics


We have developed new methods that combines the best of modern ML (end-to-end differentiable learning, flexible model) with desired statistical properties (rigorous false discovery control, sparsity, visualization). See for e.g. Nature Communications'19, Nature Communications'18, ICML'19a, ICML'19b, and AISTATS'19.
ML for new biotechnologies (spatial biology, single cells, etc.)

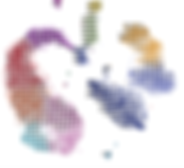
We use ML to make genome editing safer (Nature Biotech'19), to model spatial omics (Nature BME'20), to integrate single-cell multi-omics (PNAS'21), and to generate new drugs (Nature MI'19). We are excited about combining new ML with breakthroughs in genomic technologies to study human diseases (Nature Genetics'18).
Computer vision and NLP for healthcare
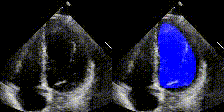

We have developed state-of-the-art computer vision algorithms for analyzing heart diseases from cardiac ultrasound videos (Nature'20), to improve telehealth (PSB,'21) and for digital pathology (Nature BME'20). We also have the best-performing NLP methods for analyzing clinical notes (Nature Digital Medicine'18, Nature Digital Medicine'19). Many of these systems are now being used by hospitals and large insurance companies.
Precision medicine for all

We pioneered methods using EHR data + AI to make clinical trials more inclusive (Nature'21) and to recommend the best treatment for cancer patients based on their mutations (Nature Medicine'22). Our work was recognized as a Top Ten Clinical Research Achievement in 2022.